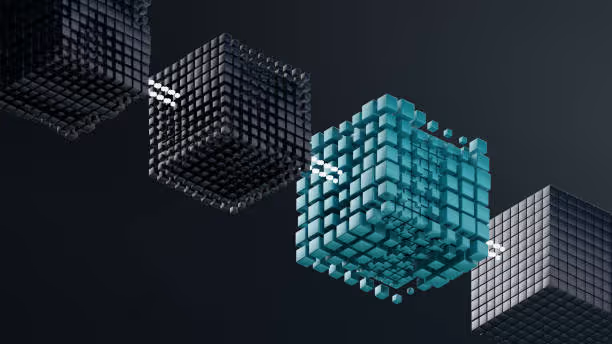
Risk Management Framework of Blockchain and DLT Systems (RMF-BDLT): Reward Mechanisms
Project Overview
As the groundwork of Risk Management Framework of Blockchain and DLT Systems (RMF-BDLT), this project focuses on monitoring, analysing and modelling blockchain reward mechanisms to manage both internal risks(inherent in protocol design) and external risks (triggered by strategic behaviour). We will develop extensiblemodelling of PoS staking, which could benefit the design and upgrade of *PoS protocols. Additionally, we willdetect anomalies such as selfish and cartel mining behaviour in real-world PoW systems and model the effectof validator’s deviation on PoS consensus.
Abstract
As the groundwork of Risk Management Framework of Blockchain and DLT Systems (RMF-BDLT), this projectfocuses on monitoring, analysing and modelling blockchain reward mechanisms to manage both internal risks(inherent in protocol design) and external risks (triggered by strategic behaviour). The team aims to develop extensiblemodelling of PoS staking, which could benefit the design and upgrade of *PoS protocols. Additionally, they willdetect anomalies such as selfish and cartel mining behaviour in real-world PoW systems and model the effectof validator’s deviation on PoS consensus.
Background
The team brings extensive expertise in blockchain and distributed ledger technologies, with several key publications addressing critical issues in the field. Their work includes a comprehensive taxonomy of blockchain technologies, analyses of centralisation in cryptocurrency platforms, and in-depth studies on the fairness of mining protocols in both proof-of-work and proof-of-stake systems. These contributions have been published in leading journals and conferences, including Ledger, IEEE, and arXiv.
The team is part of the Blockchain and Distributed Ledger Technologies (BDLT) Research Group at the University of Zurich, a leading interdisciplinary group focused on blockchain analytics, cryptoeconomics, and consensus mechanisms. Their research delves into large-scale blockchain data analysis, economic incentive structures, and agent-based modelling of blockchain protocols. The BDLT Group is recognised for pushing the boundaries of blockchain research by uncovering the mechanisms behind observed regularities in blockchain systems and exploring the dynamics of consensus protocols.
Objectives
This project aims to develop a comprehensive risk management framework for blockchain and distributed ledger technologies (RMF-BDLT), with a focus on reward mechanisms that incentivise participants and maintain system consensus. It will address both internal risks from protocol design and external risks from strategic behaviour, using empirical analysis and agent-based modelling. The project will contribute to the development of secure and fair blockchain systems by offering a framework to model staking and rewards, while also detecting and mitigating strategic attacks in Proof-of-Work (PoW) and Proof-of-Stake (PoS) protocols.
Expected Outcomes and Impact
The project will deliver an open-source framework for modelling staking and reward mechanisms across PoS systems, detecting suspicious miners in PoW systems such as Bitcoin Gold and Dogecoin. It will enhance blockchain security, ensuring stability and fairness in real-world applications. Expected benefits include increased trust within blockchain ecosystems and better decision-making for enterprises and users. This framework will serve as a key tool in advancing blockchain adoption, with findings disseminated through academic publications and conferences to further solidify its impact on the industry.
Ecosystem Development
This project aligns with the mission of restoring trust in blockchain and crypto through research by addressing selfish mining in PoW systems and promoting equitable PoS reward mechanisms. It focuses on detecting selfish mining and cartel attacks in various PoW systems, helping real-world systems detect anomalies and mitigate threats. Additionally, through the development of the RMF-BDLT, the project evaluates PoS reward incentives, analyses transaction network evolution, and measures wealth concentration and decentralisation. By providing a framework for simulating and evaluating PoS protocols, the project offers insights into how protocol parameters affect fairness and centralisation. This research enhances transparency in blockchain systems and supports more equitable reward structures, helping stakeholders make informed decisions and mitigate risks. Through its findings, the project aims to contribute to the growth, security, and adoption of blockchain technology across industries.
Methodology & Implementation
The methodology begins with the application of the blockchain taxonomy developed by Tasca and Tessone, which classifies PoS-based blockchains using components such as transaction capabilities, security, and reward mechanisms. This allows for the clustering of similar PoS systems for detailed analysis. Data collection is carried out by leveraging platform APIs and running full nodes to gather transactional, reward, and balance data, which is stored in specialised databases for further processing. For data analysis, economic and statistical metrics such as the Gini index and Nakamoto coefficient are employed to assess balance inequality and decentralisation in PoS systems, alongside methods to detect selfish mining behaviour in PoW systems. Additionally, agent-based modelling is utilised to simulate staking and reward mechanisms in various PoS protocols, helping to identify internal risks related to decentralisation and stake distribution. Throughout the project, simulations and evaluations of the impacts of protocol parameters on system security and decentralisation are conducted, with findings shared through conferences to drive further research and industry engagement.
Conclusion
This project lays the foundation for the Risk Management Framework of Blockchain and DLT Systems (RMF-BDLT) by focusing on the monitoring, analysis, and modelling of blockchain reward mechanisms. Through data-driven evaluations, the project addresses both internal risks from protocol design and external risks posed by strategic behaviours like selfish and cartel mining. By developing an extensible model for PoS staking and using agent-based modelling for PoW systems, the project aims to enhance fairness, decentralisation, and security across blockchain ecosystems, contributing to their broader adoption and long-term stability.
Progress
Materials
Project Members
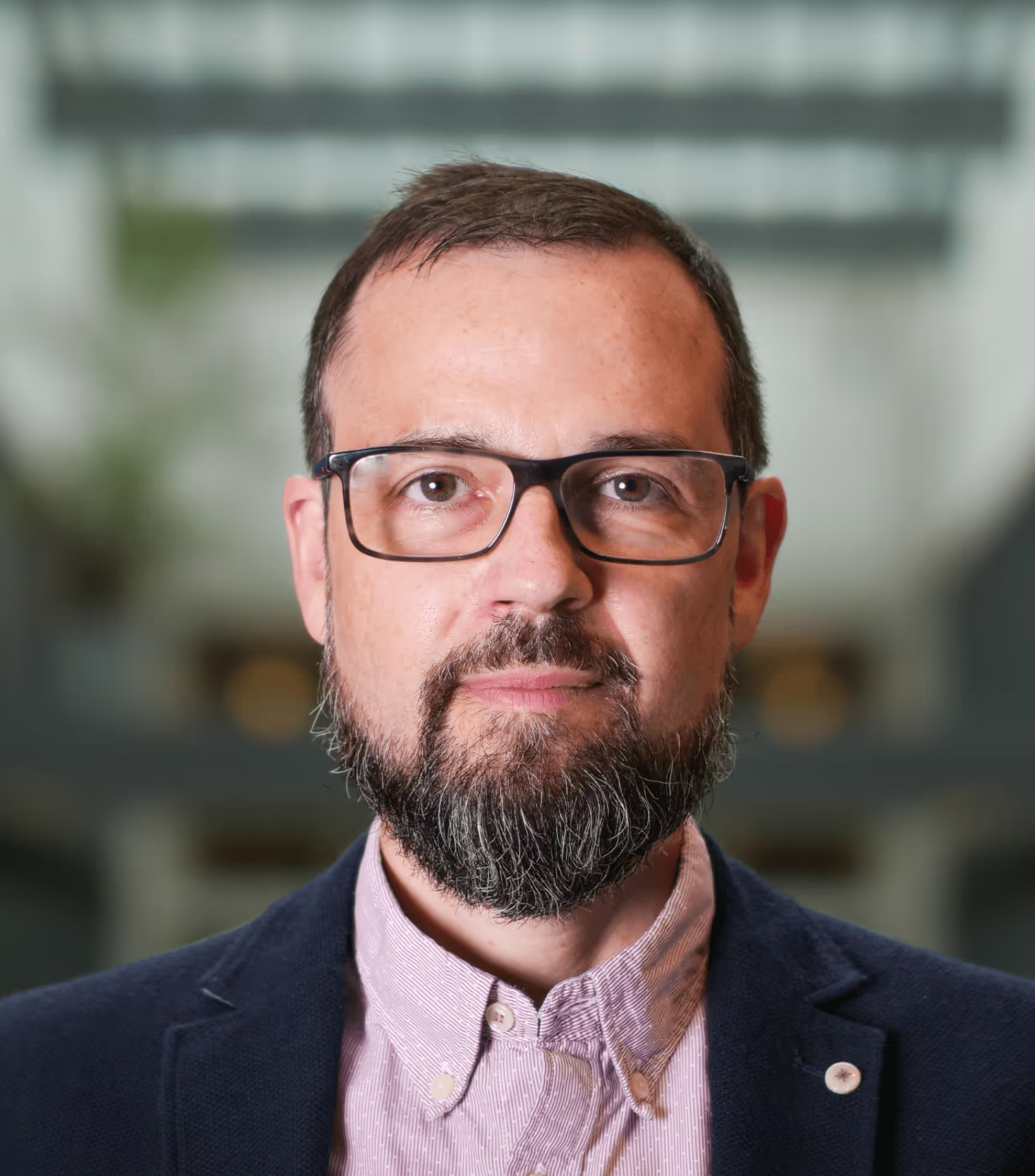
Claudio Tessone
